Reduce Customer Churn by 20% in 2 Days With Predictive Analytics and 3rd-Grade Math

Most SaaS companies have 3 misconceptions about churn:
- They don’t realize how much churn is costing them.
- They think they know why customers churn.
- They think predicting churn with data is too hard.
If you’re not using predictive analytics to prevent churn this hack will help reduce your churn by about 20%. It takes about 2 days of work over a few weeks and you can do it in Microsoft Excel. We used similar techniques to help Codeship retain 72% of their at-risk users. Download the spreadsheet to follow the example below.
You need to predict churn with data
Your customers cancel for lots of different reasons. Projects get scrapped. Users get stuck and bail. The key user takes a sabbatical to breed champion goldfish. Quite often you can intervene before this happens and prevent it - but the primary predictors of churn are not always obvious. For instance, many SaaS marketers assume last_login_at > 30 days ago predicts churn. We almost always identify better predictors such as changing patterns in user behavior. Let me re-phrase this point a little stronger: If you’re not looking at data to predict churn you are almost definitely missing the fastest, easiest way to increase your MRR.
Why this hack is effective
You don’t need a data scientist. Or the developer time.
As long as you have access to metrics in Mixpanel, Intercom, etc. even junior members of your marketing team can do it. Credit card companies invest massively in predicting churn because slight improvements generate millions of dollars. You’re not Capital One - you’re a SaaS company. You don’t need to know what “entropy” is to start predicting churn.
You don’t need statistics
Can you add? This the only math skill you need. There is one equation but we’ve already put it into the spreadsheet for you. If an addition is too complex consider outsourcing to a 3rd-grader. They’ll work for peanuts (or at least cookies).
The results are immediately actionable
We’re going to start with the data you already have in your analytics or marketing automation platform - so you can use the results to send churn-prevention emails or generate alerts for your sales team.
Step-by-Step: find the best predictors of customer churn
Download the spreadsheet
Click here to download. The examples are easier to understand if you spend a few minutes looking at the spreadsheet. I break down each step below.
PR Power! - our example company
I’m going to walk you through each step using examples from a fictitious SaaS startup called PR Power! we introduced in a previous post. PR Power! helps media managers in mid-sized businesses do better PR by generating targeted media lists. Customers pay $50-$5,000/month after a free trial. Marketing Mark, the CMO, is charged with reducing monthly churn from 5% to 4%.
Step 1 - Identify predictors of churn
Try to identify predictable reasons why customers cancel. Mark’s team spent a few hours looking at the last 20 customers who canceled and identified a few predictors. He also interviewed the sales and customer success teams about these customers. They came up with the following events that are likely to predict why a customer cancels an account with PR Power! Champion departs - Usually PR manager leaves the customer's company. Project canceled - Customer signed up for a specific PR campaign and then decides not to run the campaign.No journalists - Customer can’t find a good journalist in PR Power! to cover a story. Support fails - Customer contacts support a few times and the problem isn’t solved - usually indicated by support tickets open a long time. Stale list - Customer’s media list is less useful because journalists are no longer available or active.
Step 2 - Translate the churn predictors to data rules - or eliminate them
Mark’s team took these qualitative events and tried to identify existing data in Mixpanel that might predict them. 3 were straightforward 2 took a bit of investigating.
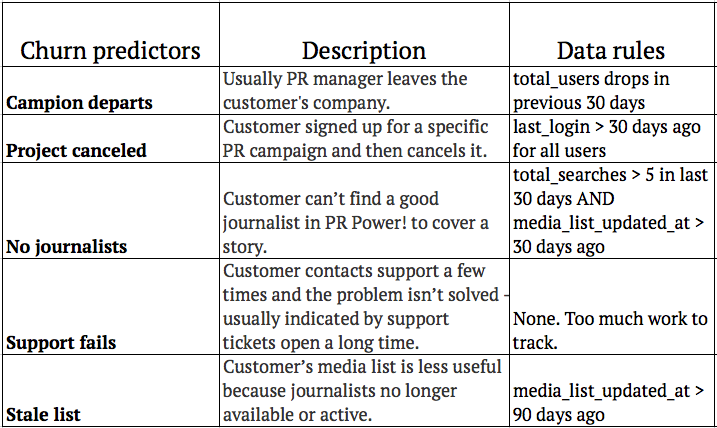
No journalists required identifying customers who had searched for journalists but didn’t add them to the media list. Support fails was simply too hard - the support desk data on tickets isn’t in Mixpanel so they decided to skip it.
Step 3 - Count the occurrences of each predictor
Mark put the predictors at the top of his spreadsheet and identified every customer who matched a data rule yesterday.
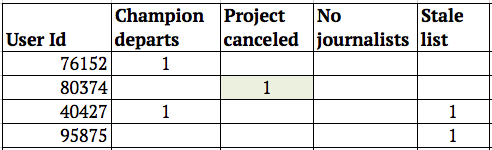
For instance, User 80374 last_login_at > 30 days ago is TRUE so he entered a 1 for Project canceled.
Step 4 - Track every customer who churns until you hit 100
Mark adds a “Canceled?” column to the spreadsheet. Each day he identifies every customer who cancels until 100 customers cancel. This takes 2 ½ weeks.

Step 5 - Count the matching events for each predictor
Now for the 3rd-grad math ...For each predictor, count every customer where the churn predictor is TRUE and the customer canceled.

Mark starts with the Project canceled rule and counts the following Number of times last_login > 30 days ago is TRUE and YES, the customer canceled.For instance, customer 80374 and 89766 fit this criteria. He counts 22 instances.
Step 6 - Enter the results into the spreadsheet
Enter the total in the appropriate block of the 3x3 matrix to calculate the Prediction Score (This is implementation of the Phi coefficient). Mark enters 22 and calculates Prediction Score for Project canceled at 0.009

Step 7 - Identify the biggest predictors of churn
Rules with the higher Prediction Score are better predictors of churn.Mark compares the Prediction Score for each rule and sees an obvious pattern.
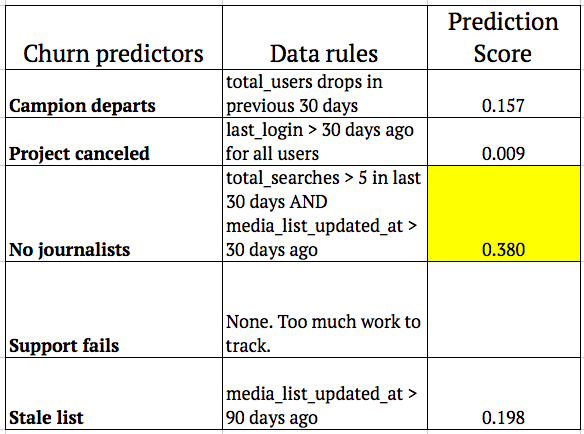
Two observations immediately jump out at Mark: First, last_login_at > 30 days ago doesn’t tell him much about Project canceled. Since PR Power! has long-term customers who use the product periodically this isn’t surprising. Second, No journalists are the clear winner. In hindsight, this makes sense - customers who try to find a journalist and can’t are getting no value from the product.
Step 8 - Take steps to prevent churn
Mark creates 2 rules in Mixpanel for the No journalists predictor.
Small accounts
When a customer has total_searches > 5 within the last 30 days AND media_list_updated_at > 30 days ago Mark creates an auto-message inviting a customer to watch a webinar on “How to search for a journalist”.
Large Accounts
When a customer has total_searches > 5 within the last 30 days AND media_list_updated_at > 30 days ago Mark creates an alert for the sales team to notify them about a customer at risk for churning.
An easier way - ask us to do this for you
You don’t need even need 3rd-grade math. Just take a free trial of MadKudu and let us run these calculations for you. Cancel anytime if you don’t like it - keep whatever you learn and all the money you make from reducing your churn. Want to learn more? Sign up for our new course. Photo credit: Rodger Evans