Onboarding Growth Experiments for Your Worst Users

Understanding who your product is best suited for is critical. If you know what your best leads look like and how they behave, you can design an ideal buyer journey for them, making sure that anyone who looks like your best leads stays on the buyer journey that your best leads take. That said, like all channels for optimization, you eventually hit diminishing returns. The major holes get filled, and your customer journey works 95% as well as it ever well. What's worse, by only focusing on creating a great experience for leads who look like leads who have historically converted well, you may create a self-fulfilling prophecy. If you're only a good fit for your current ICP, you may never be a good fit for the ICPs you want to be targeting in the future. We see this up close at MadKudu. Since predictive lead scoring models leverage your historical customer data to predict the likelihood that future prospects will convert, if you don't feed it new data such as successful conversions from leads who historically haven't converted well, then your ideal customer profile will never change. Product growth from a predictive modeling perspective can be framed as an exercise in feeding new "training data" that the model can later use to adapt and expand the definition of your ICP, your best leads.If your product is only accessible via the United States because it requires a US bank account, address or phone number for authentication, leads from outside the U.S. will have a low likelihood of converting. If you build new features or expand into new markets but continue to score leads with old data, you may not give new leads a chance to have a great experience. Growth (for low-touch) & business development (for high-touch) are great teams for circumventing this process, and many MadKudu customers leverage these teams to create new training data by actively pursuing leads who haven't historically converted well, but that the business would like to target. This can be expanding into new verticals, new markets, or launching new products altogether. All three are areas where historical customer data isn't a great basis for predicting success, because the aim is to create success that can later be operationalized, orchestrated and scaled.
Parallel onboarding for good & bad leads.
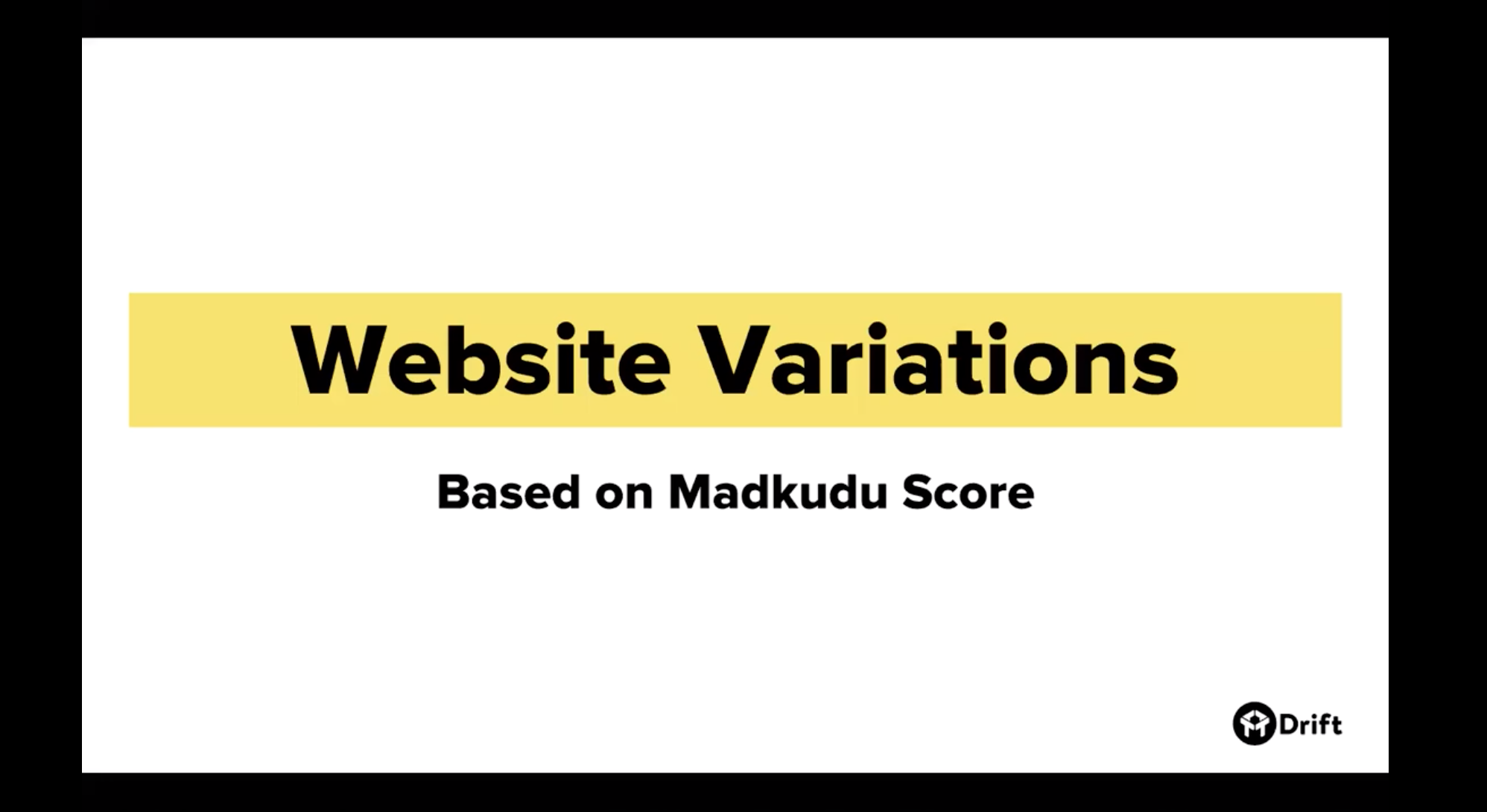
Drift recently gave a talk during the Product-Led Summit about a series of onboarding experiments that segmented their best & worst leads, but that pushed to increase conversion among both segments. Highlighting some of their experiments, it is clear that Drift's aim was almost to prove the model wrong - that is, they wanted to optimize the chances that a low probability lead would convert, which later could help to retrain the definition of a good/bad lead.
Leads: good vs. bad
Good leads are those who are most likely to convert. In sales-driven growth companies, that means that, if engaged by velocity/enterprise sales, a lead will convert. For product-led growth companies with no-touch models, a good lead is one that is likely to achieve is a certain MRR threshold, if activated. We define good leads this way - instead of, say, the type of leads we want to convert - because we want to create as much velocity & efficiency for our growth-leading teams as possible. If we send a lead to sales that won't convert no matter how much we want to, we are incurring an operating cost associated to that rep's time. That counts double for leads that will convert the same amount whether engaged by sales or not, as we are unnecessarily paying out commission. Product teams focused on lead activation & conversion waste time running experiments with little to no impact if they don't properly segment between good & bad leads.
Drift's 5 Growth Experiments segmented by customer fit
Drift used MadKudu to segment their entire onboarding & activation experience so that good leads and bad leads each received the best next action at each step of their buyer journey.
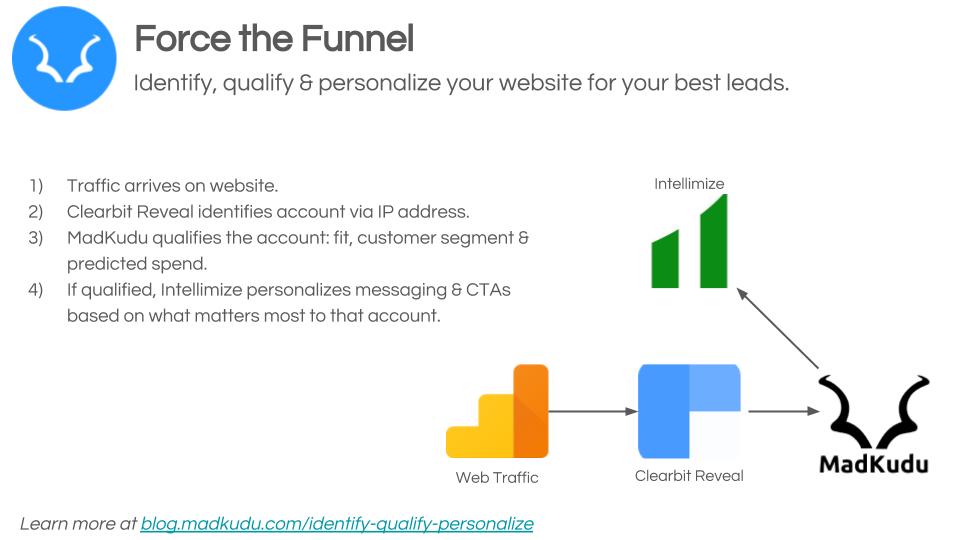
For Drift, onboarding starts before users create an account. Drift Forces the Funnel by identifying website visitors via IP lookup as they arrive on their website, scoring the account associated to the IP, and then personalizing the website experience based on the MadKudu score and segment. For their best leads, Drift's messaging is optimized to provide social proof with customer logos & key figures with a core call-to-action to talk with someone. Drift is willing to invest SDR resources in having conversations with high-quality leads because they convert more consistently and at MRR amounts that justify the investment. For their worst leads - that is, leads that won't convert if engaged by sales - Drift's messaging is tailored towards creating an account and "self-activating," as we'll see in future experiments.
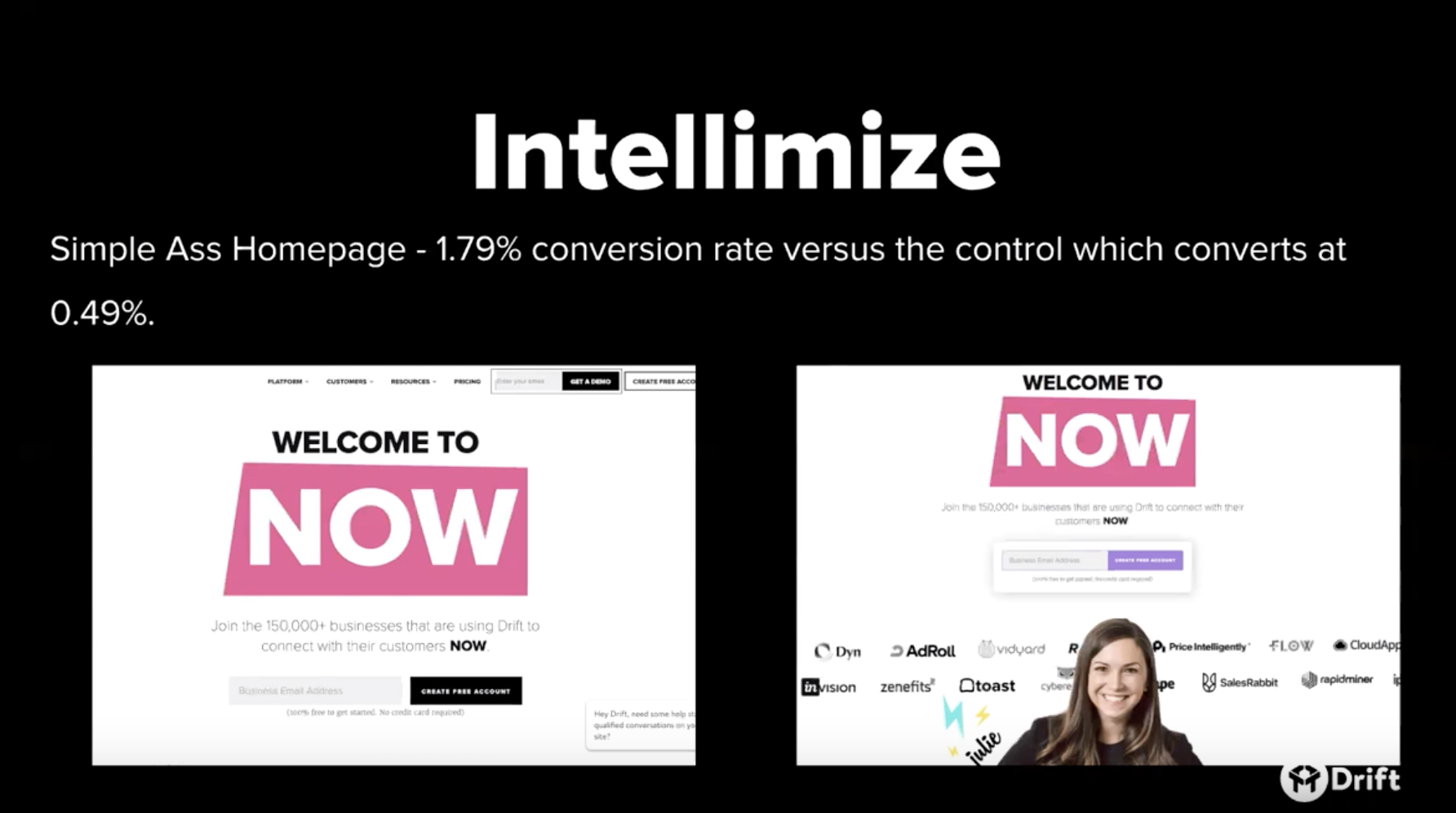
For major sources of traffic, like their pricing page or the landing page for website visitors who click on the link inside their widget for free users, Drift iterates constantly on how to improve conversion. Some experiments work, like stripping out the noise for low-quality leads to encourage self-activation. Others, such as dropping a chatbot inside the onboarding experience for high-quality leads, don't get as much traction despite good intentions & hope.
Intentional Onboarding
Sara Pion spent a good amount of time praising the impact of onboarding questions that allow users to self-identify intent. User inputed fields can be very tricky - mainly because users lie - but Drift has found a heavy correlation between successful onboarding and the objective that the user declared they had when signing up. As users onboard, good & bad users are unknowingly being nudged in two very different directions. Emails, calls to action, and language for good leads are geared towards speaking with a representative. That's because Drift knows that good users who talk with someone are more likely to convert. Bad users, meanwhile, are encouraged to self-activate. Onboarding emails encourage them to deploy Drift to their website, to use certain features, and generally to do the work themselves. Again, that's because Drift knows that talking to these users won't statistically help them be successful - either because they don't actually need Drift or because they want to use Drift their own way without talking to someone.
Personalize the definition of success for every user
Like most successful SaaS companies, Drift has invested an awful lot of energy making sure that their best leads have the best possible buyer journey; however, unlike most companies, they don't stop there. They look at how they can optimize the experience for their worst leads as well, recognizing that even a 1% increase in conversion can be the difference between hitting their revenue goals or not given the massive volume of leads they get each month.
https://www.youtube.com/watch?v=LRb5_Rzzb9U